Date:
By Iosif Itkin, CEO & Сo-Founder, Exactpro
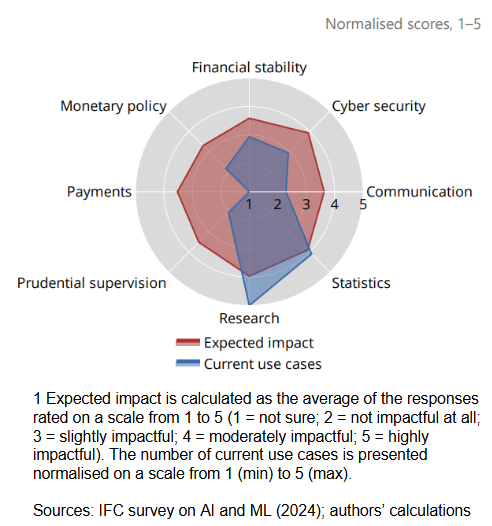
Personal use of AI in the workplace is widespread across industries, and the financial space is no exception. Yet, its impact on the organisations' bottom lines is still either non-existent or extremely hard to track or formally attribute – a sign of the Productivity Paradox.1 If evolving alongside trajectories similar to the ones taken by other general-purpose technologies like the information technology itself, AI is yet to see the necessary ratio of derivative innovations springing off it, before quantifiable, i.e. enterprise- or national-level, gains in productivity can be assigned to it. The recent IFC Report on Governance and implementation of artificial intelligence in central banks states that, “despite high expectations, current AI-based applications [in central banks] remain limited (illustrated in Fig.1) and primarily relate to economic research, communication chatbots and statistics.”2 Within the regulated space, widespread AI implementation remains hampered by a skills shortage, as well as scalability, safety, ethics, compliance, integration, and other concerns that are yet to be resolved.
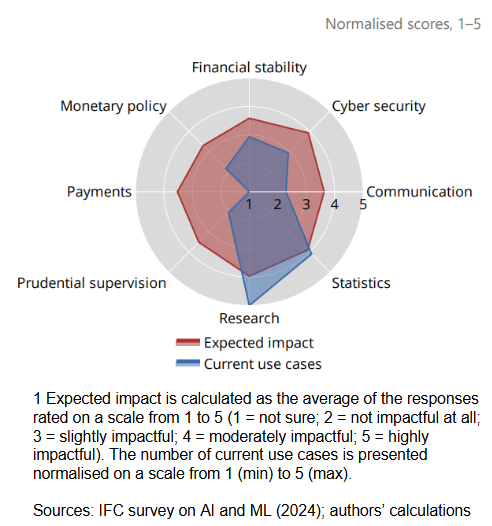
Should organisations postpone substantial investments until more reliable AI technology comes around, or are the fears of missing out well-justified? There are several reasons why laying an AI foundation within your organisation could be a prudent strategy for the challenges ahead. First of all, we live in an “exponential age,”3 and technologies tend to take significantly less than expected to mature and expand. Secondly, the convergence of factors such as the growth of available digital data volumes and computational capabilities, as well as competitive pressure from other industries and use cases, makes now a strategic moment to move towards actively preparing for AI adoption.
Time and effort are required to shape the internal AI culture, develop in-house expertise and coordination workflows, and set governance practices. Failing to prepare the data ecosystem and the workforce may later result in rushed and risk-prone deployments, misaligned workflows or implementations.
Investing in AI preparedness allows organisations to:
- Gain a competitive advantage via creating an AI-ready environment: embedding pervasive internal AI literacy into the workforce, training all business and technical staff early.
- Drive holistic change: platforms will only be an infrastructure constituent – upcoming changes in supporting workflows and processes, security, and people need to be factored in (illustrated in Fig.2, as per the Zero Outage Industry Standard (ZOIS)4).
- Set up governance early, developing ethics, data, testing, security, and compliance principles and frameworks that should not be afterthoughts.
- Start small with pilots in high-impact, low-risk areas.
Fig.2 The People, Processes, Platforms, and Security pillars of the Zero Outage Industry Standard.
AI Adoption in Financial Organisations: Strategic Advice
To be able to propel innovation across all business lines in the near future, building a solid foundation in AI literacy and expertise now is key. AI literacy is also one of the main four focus areas proposed by The Bank of International Settlements in the report cited above.5 One way to start is engaging in industry collaborations and knowledge transfers with organisations specialising in AI training. Another way is exploring AI applications via PoC-level engagements with technology vendors, for whom innovation may be a more dynamic process. Sandbox experimentation and education foster top-tier talent and bring to life the necessary cultural changes. They also allow organisations to understand the AI momentum and safely explore and assess the value of AI case-by-case, whilst building the surplus of in-house knowledge necessary for internal innovations to take off.
Financial & Self-learning Systems: Shared Characteristics
According to the International Software Testing Qualifications Board (ISTQB®)6 classification, the challenges inherent to self-learning systems are summarised as: unexpected change, complex acceptance criteria, insufficient testing time, resource requirements, insufficient specifications of operational environments, complex test environment, bias of data inputs, and undesirable behavior modifications. These are identical to the challenges faced when assessing the output quality and the performance of distributed financial platforms built with traditional technologies. This is due to the fact that the two types of systems share many characteristics that result in their non-determinism and intrinsic complexity.
A comprehensive quality assessment approach to both types of systems calls for holistic multi-layered test strategies. Understanding the depth, breadth, and sequence of actions behind a structured quality assessment framework prepares an organization for adopting a high-quality architecture from the outset.
Robust Quality Assessment – A Gateway to AI Adoption
In the high-risk, compliance-heavy environments where financial systems operate, the role of testing as an information gathering tool is sometimes overlooked. Formulating a robust test strategy – that is also adaptable to foreseeable product evolution or requirements changes – helps prevent potential negative factors and defects early, well before they can occur further in the lifecycle, and formulate all stakeholder needs well ahead of the last development stages. The test strategy defined during a collaboration can provide an industry-tested software transformation framework transferable to client’s projects of any scale.
Furthermore, organisations can benefit from time-boxed pilot test engagements in AI Testing that enable: practical, hands-on exposure to AI in an isolated segment of their infrastructure – quality assurance – introducing internal QA, DevOps, and engineering teams to real-world AI usage. This involves AI-enabled test ideas generation, defect analysis and prediction, results analysis, continuous models improvement – all performed in a controlled environment without needing to build internal AI capabilities from the ground up. Organisations can obtain fast feedback on value and risks across a set of criteria including software testing speed, costs, quality of the information obtained about the system under test, and quality of the models involved in testing, whilst seeing measurable outcomes to support or adjust broader AI strategies.
Pilot trials can increase trust and internal buy-in, reducing AI resistance via safe and well-contained experimentation. Last but not least, this incremental step fosters the development of reusable and scalable governance practices aligned with future AI initiatives (e.g., ISO 42001). A time-boxed AI testing partnership acts like a pilot project that delivers value fast, builds foundational knowledge, and reduces the perceived risk of adopting AI organization-wide.
AI Training
As a technology vendor, we support the quality aspects of some of the industry’s major FMI transformation initiatives, which makes us keep our own technology and practices to the highest standards. Built on vast industry experience, our capabilities in efficient knowledge transfers and promoting professional excellence come as second nature.
Exactpro’s ISTQB®-accredited AI Testing course7 has been bringing value to individual and corporate participants. The course can be used to augment in-house AI literacy capabilities and equip teams with foundational AI knowledge via a variety of interactive reading materials, workshops, discussions, and practice tests. The topics touched upon in the course include but are not limited to data processing, ML model development and deployment, test design, using AI to automate workflows, streamline synthetic data generation and data analysis tasks, enhancing the efficiency of test coverage and helping improve system robustness via better defect detection.
I am delighted to be on the team catalysing a truly transformative shift and sharing cutting-edge future-ready techniques to ensure the quality and resilience in exchanges, clearing houses, securities depositories, payment platforms, and other financial market infrastructures. I look forward to discussing collaboration opportunities with the WFE Members, Affiliates and Partners to make our industry better-prepared for the risky – but rewarding – journey ahead.
References
- Butler, A. ( 2025) The AI Productivity Paradox? Available at: https://abutler.com/the-ai-productivity-paradox/ (Accessed 25.04.2025)
- Bank for International Settlements (2025). ‘2. High interest but still low adoption.’ IFC Report No 18 Governance and implementation of artificial intelligence in central banks, p 7. Available at: https://www.bis.org/ifc/publ/ifc_report_18.pdf (Accessed 29.04.2025)
- Azhar, A. (2024) The Exponential View, Five charts to understand the Exponential Age. Available at: https://www.exponentialview.co/p/five-charts-to-understand-the-exponent… (Accessed 25.04.2025)
- Zero Outage Industry Standard (2025). Architecture. Available at: https://zero-outage.com/the-standard/architecture/
- Bank for International Settlements (2025). ‘5. A roadmap to promote innovation in the evolving data and technological landscape.’ IFC Report No 18 Governance and implementation of artificial intelligence in central banks, p 20. Available at: https://www.bis.org/ifc/publ/ifc_report_18.pdf (Accessed 29.04.2025)
- Klaudia Dussa-Zieger et al. (2021) ‘Challenges Testing Self-Learning Systems.’ ‘Certified Tester AI Testing (CT-AI) Syllabus.’ ISTQB®,Version 1.0, pp 58-59. Available at: https://www.istqb.org/wp-content/uploads/2024/11/ISTQB_CT-AI_Syllabus_v… (Accessed 25.04.2025)
- Exactpro (2025). ISTQB®-accredited AI Testing Training course. Accessible in Autonomous, Guided, Accelerated and Enterprise plans. Available at: https://exactpro.com/training/ISTQB-AI-Testing-course